ProWD: Detecting Knowledge Imbalances on Wikidata
Elisabeth Giesemann
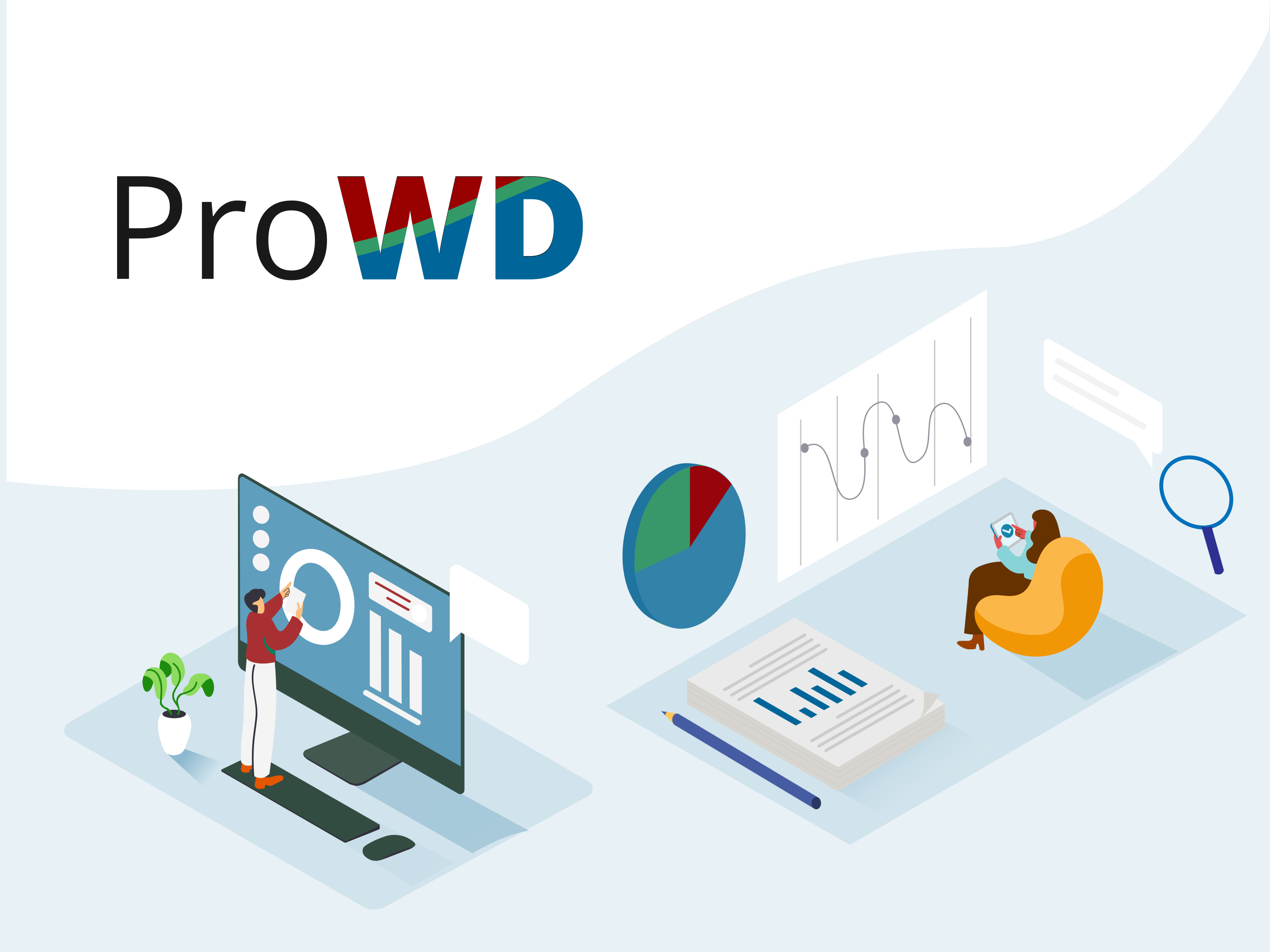
With 89 million Items as of this writing, Wikidata is the largest open database in the world. Yet it still has gaps and imbalances in its data. The reasons for the imbalances are complex: historically, large amounts of data from the fields of natural science and Western history have been collected for a long time, while other areas, such as non-Western cultures and minorities, are systematically underrepresented in literature and science. To highlight these gaps, ProWD analyzes Wikidata’s distribution of data. By creating dashboards for a topic, users can discover these gaps and remedy them.
Elisabeth Giesemann: Tell us a little about who you are and how you discovered ProWD.
Nadyah Hani: We are both students of Computer Science at Universitas Indonesia. I’m interested in Data Analytics and User Research and I also do Front-end Development.
Refo Ilmiya: I’m mostly interested in data science and software engineering. We discovered the project in a class on the semantic web and joined the research team. It was a great learning opportunity; this month we graduated from our bachelor program.
Elisabeth: What was it like to use Wikidata for a university project?
Nadyah: ProWD is an iteration of a research project. It was initially created by a senior student, Avicenna Wisesa, and we developed it further. We worked on it for six months; the first two months we studied Wikidata and the Semantic Web, then for four months we developed it, with help from Dr. Fariz Darari and Dr. Panca O. Hadi Putra from Universitas Indonesia, as well as Prof. Werner Nutt from the Free University of Bozen Bolzano and Dr. Simon Rasniewski from the Max Planck Institute.
Refo: We both had little prior experience with Wikidata, and we weren’t active users until the research project. Two years ago, we went to a workshop at our university whose subject was data about Indonesia on Wikidata. It was mostly an orientation on how to access Wikidata and contribute data.
Elisabeth: Please explain ProWD and how it works.
Nadyah: ProWD shows the distribution of entries among a class of Wikidata Items. To do this, it uses the measure of the Gini coefficient, which measures inequality among values of a frequency distribution and is mostly known for describing levels of income inequality. A Gini coefficient of 0 represents perfect equality, where all values are the same — for example, where everyone has the same income. A Gini coefficient of 1 (or 100%) expresses maximal inequality among values.
Refo: If you’re interested in a topic on Wikidata, you can profile it by filtering it. Select the class and filters for your topic of interest, and you can identify underrepresented topics. ProWD shows you the Gini coefficient and the distribution of Wikidata Items for your topics. This brings the user one step closer to adding data where it’s missing.
Nadyah: The app also has dashboards to play around with if you want to try it out first.
Refo: Basically, ProWD shows you whether a class of Wikidata Items has an equal number of Properties. What was surprising was that most classes of Items are already fairly balanced. However, subclasses often have high imbalances.
With ProWD you can filter people in Wikidata who have the occupation of Computer Scientist. We see that the class is imbalanced, which means that some Items in the class have many entries in Wikidata, while others do not.
The tool allows the user to compare the regional distribution of Computer Scientists worldwide.
The tool also provides an overview of the gender distribution of computer scientists who have an entry in Wikidata.
Elisabeth: How does this solve imbalances on Wikidata?
Nadyah: Once you’ve detected a class that is heavily imbalanced, you can look up the most common Properties on ProWD, see which Items might lack a popular Property and start adding them. This way you can slowly close the gaps between the Items in that class.
Refo: The app can be used for anomaly detection and comparison. By shedding light on gaps in the data, it can trigger further investigations on existing why the imbalances exist. Here, ProWD mainly functions as a detection system.
Nadyah: The data can give you a first impression, after which you can analyze the reason for the imbalance. Looking at the data can lead to more research and reflection, e.g., we need to pay more attention to gender distribution among scientists in real life.
Elisabeth: What do you recommend for other developers who want to build tools with Wikidata?
Nadyah: Reach out to your user base early and focus on their needs. Instead of how something can work technologically, I think it’s important to understand what Wikidata users really need to improve data quality and then make it available to new users. The Wikimedia community in Indonesia was very helpful; they took part in interviews to test the usability. But it would be amazing if we could one day do user research with the international community.
Elisabeth: What’s ProWD’s next step?
Refo: Now that we’ve graduated from our programs, we plan to submit papers in which we describe both the analytics and the interface.
Nadyah: There is still a lot of potential in Open Data that we can tap into with the right tools. For ProWD, other students will work on it and improve it even further.
Nadyah and Refo show me the most interesting WIkidata classes using their university project ProWD.
No comments yet
Leave a comment